Machine learning helps organizations make valuable predictions from data. This is by training models to make data analysis and drawing useful insights from it easy. But then, this sometimes doesn’t happen because of the various challenges that ML mo+dels face.
You must be able to identify these problems in time and solve them. This is what this article looks to discuss. It will help you find ways to identify issues in machine learning models. Read to the end to know how to identify these problems effortlessly.
Let’s get started.
Model Management
As mentioned earlier, models need to deliver value and provide a competitive advantage. It is therefore vital that you ensure your models do this consistently. And, the best way to do that is by properly managing them. Proper model management helps you identify issues and address them.
Identifying issues in machine learning models may be hard to do with just the naked eye. And that is where model management comes in handy. However, machine learning model management is a broad category that encompasses a lot of tasks that businesses have to do.
Model Management starts with checking if you have the proper infrastructure. It then goes further to help ensure you develop high-quality models. You can also check your production, governance, and context. All these play a role in ensuring high ML model quality.
You need the skills to properly manage your ML models. But then, it is also possible to do machine learning model management without the necessary skill set. All you need to do is outsource it to experts. It will help maintain your models and ensure they deliver to expectations.
Model Monitoring
Models are different in various aspects. For instance, they are built differently, use varying materials and behave differently. Therefore, you cannot treat all your models the same way. If you know this and implement it, it will be easier to unlock the full potential of your models.
Model monitoring is a process that’s necessary. It makes it easy for an organization to monitor model performance after deployment. Model monitoring aims to ensure that models remain accurate and provide quality predictions.
Proper model management helps get generalization out of the way. This is key because it ensures stability in the predictions, which is good for a business. Monitoring also makes it easy for a company to analyze its data and ensure data integrity in the process.
It is also not easy to run continuous business operations without model monitoring. A business that monitors its models also gets to manage its finances better. All these are key to ensuring the continuity of your business operations, making them not worth overlooking in a business.
You can use model monitoring tools to make this process seamless. These tools can increase the efficiency and productivity of your teams. But then, you should start by researching tools that will bring the desired value to you. Various factors can help you do that effortlessly.
For instance, ensure the tools you pick allow easy integration with model monitoring. It is also vital that they offer altering capabilities and monitoring features. Last but not least, ensure the tools have a user-friendly interface and have application programming interfaces.
This can make it easy for you to get the desired value for your business. In the end, your business will be highly profitable, and achieving your goals will be easier. Also, you’ll quickly know the problems in your machine models and solve them for business continuity.
Common ML Model Issues to Identify and Solve
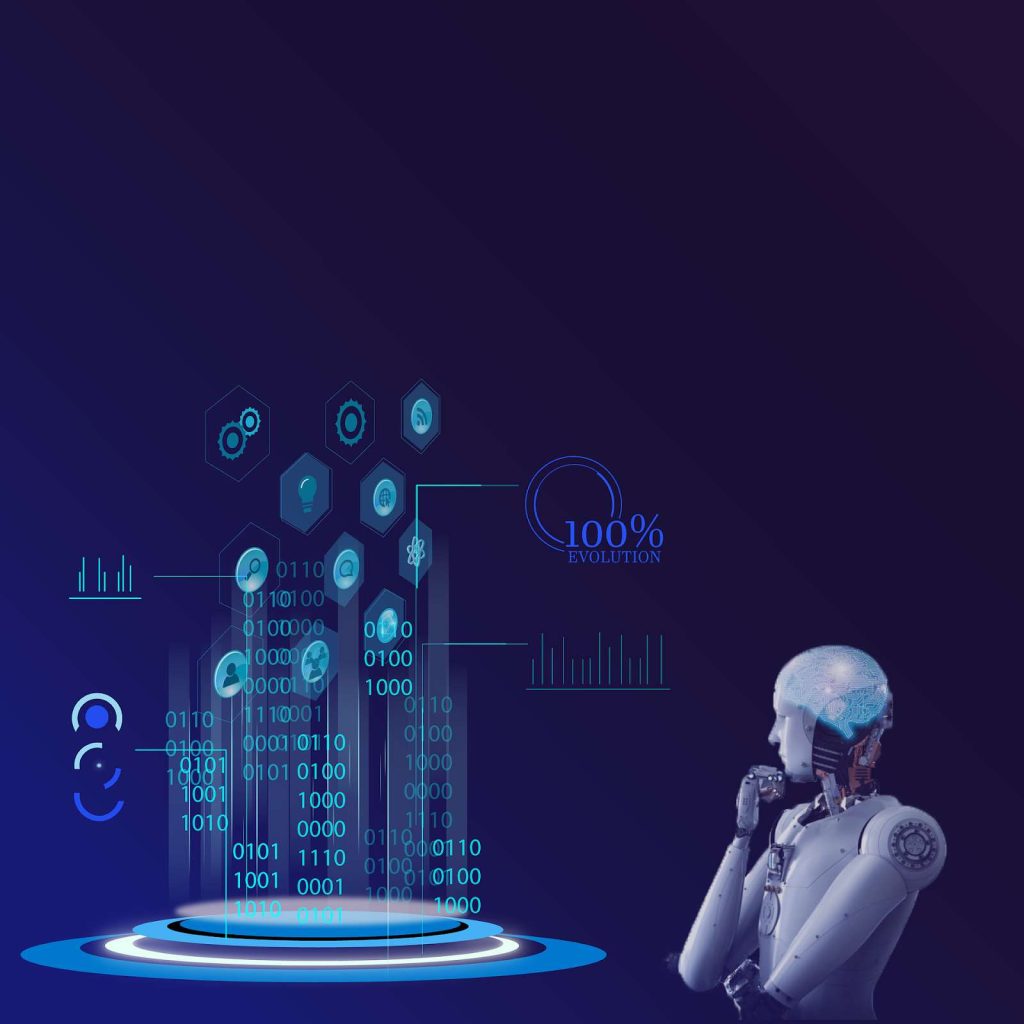
Since machine learning models are products of technology, continuous changes should be adapted to improve their functionality. In addition, these innovations are not perfect and will most likely have errors in the long run.
Knowing what to look out for is one factor in ensuring you solve them effectively. Once solved, it will be easier for your models to provide accurate predictions. Keeping a smoothly running and error-free model helps provide better results. It will also be easier for your teams to deliver to your expectations.
Here are some problems:
- It can be tricky to understand the areas of your business that are worth automating.
- Lack of good data. The data quality determines how accurate the predictions made using models will be.
- There could be inadequate infrastructure to handle the available workload and deliver results under pressure.
- It can be challenging to implement services, find anomalies, and ensure accurate predictive analysis.
- Lack of skilled resources such as employees who can manage and develop analytical ML content.
Effectively solving these problems can help a business significantly. It will make it easy to optimize the capabilities of machine learning. In the end, taking your business to the next level will be almost effortless. Model monitoring requires tracking and observation, which is relatively easy.
Combining model monitoring and management can bring the desired results to a business. All you need to do is ensure you are doing everything correctly. Thankfully, you do not need special skills to do this effectively. All you need to do is find the right tools for your work.
As mentioned before, there are model monitoring tools that can be helpful in this. For model management, consider working with an expert for the best results. They’ll help ensure you do everything right and get the desired results in the end.
Conclusion
Now you know how to identify issues in your ML models. But it would be best if you didn’t stop after identifying these ML model issues. It would help if you acted upon them to ensure your ML models provide the desired value to your business. For instance, the problem with your ML models could be a lack of quality data.
If data is the problem, take your time to evaluate it. Consider putting more data governance, integration, and exploration. These will help enhance the quality of your data. In the end, this will help make your MLmoidels more effective and helpful in making predictions for the future.
Apart from this, if you are interested to know about “Use GNOME on CentOS” then visit our Business category.